diff --git a/README_Unity.md b/README_Unity.md
deleted file mode 100644
index 5f6b8831..00000000
--- a/README_Unity.md
+++ /dev/null
@@ -1,28 +0,0 @@
-# Generating files for Unity rendering
-
-This readme contains guidances for generating files required for Unity rendering in my [Unity project](https://github.com/kwea123/nerf_Unity)
-
-## MeshRender
-
-See [README_mesh](README_mesh.md) for generating mesh.
-You then need [this plugin](https://github.com/kwea123/Pcx) to import `.ply` files into Unity.
-
-## MixedReality
-
-Use `eval.py` with `--save_depth --depth_format bytes`to create the whole sequence of moving views. E.g.
-```
-python eval.py \
- --root_dir $BLENDER \
- --dataset_name blender --scene_name lego \
- --img_wh 400 400 --N_importance 64 --ckpt_path $CKPT_PATH \
- --save_depth --depth_format bytes
-```
-You will get `*.png` files and corresponding `depth_*` files. Now import the image you want to show and its corresponding depth file into Unity, and replace the files in my Unity project.
-
-## VolumeRender
-
-Use `extract_mesh.ipynb` (not `extract_color_mesh.py`!) to find the tight bounds for the object as for mesh generation (See [this video](https://www.youtube.com/watch?v=t06qu-gXrxA&t=1355)), but this time stop before the cell "Extract colored mesh". Remember to set `N=512` in the cell "Search for tight bounds of the object" and comment out the lines for visualization. Now run the cell "Generate .vol file for volume rendering in Unity", after that, you should obtain a `.vol` file, which you can import to my Unity project and render.
-
-**NOTE:** If you use colab as in the video, copy the cell "Generate .vol file for volume rendering in Unity" into colab notebook and execute it.
-
-If you want to render in your own project, you need the script [LoadVolume.cs](https://github.com/kwea123/nerf_Unity/blob/master/Assets/Editor/LoadVolume.cs) which reads this own-defined `.vol` into a `Texture3D`.
diff --git a/README_mesh.md b/README_mesh.md
deleted file mode 100644
index 9842141f..00000000
--- a/README_mesh.md
+++ /dev/null
@@ -1,66 +0,0 @@
-# Reconstruct mesh
-
-Use `extract_mesh.ipynb` (the notebook, **not** the py script) to extract **colored** mesh. The guideline for choosing good parameters is commented in the notebook.
-Here, I'll give detailed explanation of how it works. There is also a [video](https://youtu.be/t06qu-gXrxA) that explains the same thing.
-
-## Step 1. Predict occupancy
-
-As the [original repo](https://github.com/bmild/nerf/blob/master/extract_mesh.ipynb), we need to first infer which locations are occupied by the object. This is done by first create a grid volume in the form of a cuboid covering the whole object, then use the nerf model to predict whether a cell is occupied or not. This is the main reason why mesh construction is only available for 360 inward-facing scenes as forward facing scenes would require a **huge** volume to cover the whole space! It is computationally impossible to predict the occupancy for all cells.
-
-## Step 2. Perform marching cube algorithm
-
-After we know which cells are occupied, we can use [marching cube algorithm](https://en.wikipedia.org/wiki/Marching_cubes) to extract mesh. This mesh will only contain vertices and faces, if you don't require color, you can stop here and export the mesh. Until here, the code is the same as the original repo.
-
-## Step 3. Remove noise
-
-The mesh might contain some noise, which could be due to wrongly predicted occupancy in step 1, or you might consider the floor as noise. To remove these noises, we use a simple method: only keep the largest cluster. We cluster the triangles into groups (two triangles are in the same group if they are connected), and only keep the biggest one. After removing the noise, we then compute the color for each vertex.
-
-## Step 4. Compute color for each vertex
-
-We adopt the concept of assigning colors to vertices instead of faces (they are actually somehow equivalent, as you can think of the color of vertices as the average color of neighboring faces and vice versa). To compute the color of a vertex, we leverage the **training images**: we project this vertex onto the training images to get its rgb values, then average these values as its final color. Notice that the projected pixel coordinates are floating numbers, and we use *bilinear interpolation* as its rgb value.
-
-This process might seem correct at first sight, however, this is what we'll get:
-
-
-
-by projecting the vertices onto this input image:
-
-
-
-You'll notice the face appears on the mantle. Why is that? It is because of **occlusion**.
-
-From the input image view, that spurious part of the mantle is actually occluded (blocked) by the face, so in reality we **shouldn't** assign color to it, but the above process assigns it the same color as the face because those vertices are projected onto the face (in pixel coordinate) as well!
-
-So the problem becomes: How do we correctly infer occlusion information, to know which vertices shouldn't be assigned colors? I tried two methods, where the first turns out to not work well:
-
-1. Use depth information
-
- The first intuitive way is to leverage vertices' depths (which is obtained when projecting vertices onto image plane): if two (or more) vertices are projected onto the **same** pixel coordinates, then only the nearest vertex will be assigned color, the rest remains untouched. However, this method won't work since no any two pixels will be projected onto the exact same location! As we mentioned earlier, the pixel coordinates are floating numbers, so it is impossible for they to be exactly the same. If we round the numbers to integers (which I tried as well), then this method works, but with still a lot of misclassified (occluded/non occluded) vertices in my experiments.
-
-2. Leverage NeRF model
-
- What I find a intelligent way to infer occlusion is by using NeRF model. Recall that nerf model can estimate the opacity (or density) along a ray path (the following figure c):
- 
- We can leverage that information to tell if a vertex is occluded or not. More concretely, we form rays originating from the camera origin, destinating (ending) at the vertices, and compute the total opacity along these rays. If a vertex is not occluded, the opacity will be small; otherwise, the value will be large, meaning that something lies between the vertex and the camera.
-
- After applying this method, this is what we get (by projecting the vertices onto the input view as above):
-
-
- The spurious face on the mantle disappears, and the colored pixels are almost exactly the ones we can observe from the image. By default we set the vertices to be all black, so a black vertex means it's occluded in this view, but will be assigned color when we change to other views.
-
-
-# Finally...
-
-This is the final result:
-
-
-
-We can then export this `.ply` file to any other format, and embed in programs like I did in Unity:
-(I use [this plugin](https://github.com/kwea123/Pcx) to import `.ply` file to unity, and made some modifications so that it can also read mesh triangles, not only the points)
-
-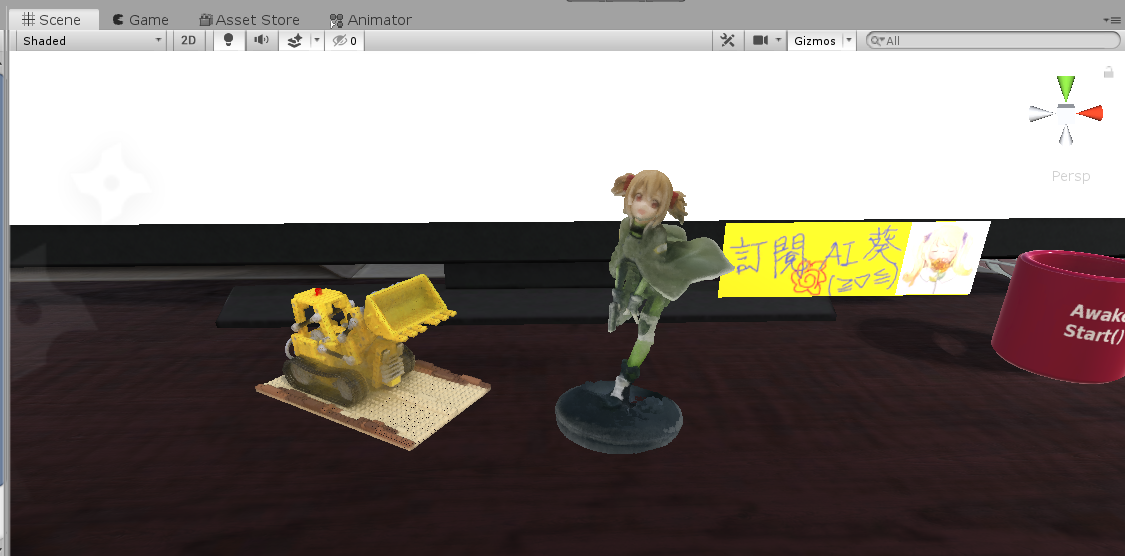
-
-The meshes can be attached a meshcollider so that they can interact with other objects. You can see [this video](https://youtu.be/I2M0xhnrBos) for a demo.
-
-## Further reading
-The author suggested [another way](https://github.com/bmild/nerf/issues/44#issuecomment-622961303) to extract color, in my experiments it doesn't turn out to be good, but the idea is reasonable and interesting. You can also test this by setting `--use_vertex_normal`.
diff --git a/extract_color_mesh.py b/extract_color_mesh.py
deleted file mode 100644
index 114d3b9b..00000000
--- a/extract_color_mesh.py
+++ /dev/null
@@ -1,297 +0,0 @@
-import torch
-import os
-import numpy as np
-import cv2
-from collections import defaultdict
-from tqdm import tqdm
-import mcubes
-import open3d as o3d
-from plyfile import PlyData, PlyElement
-from argparse import ArgumentParser
-
-from models.rendering import *
-from models.nerf import *
-
-from utils import load_ckpt
-
-from datasets import dataset_dict
-
-torch.backends.cudnn.benchmark = True
-
-def get_opts():
- parser = ArgumentParser()
- parser.add_argument('--root_dir', type=str,
- default='/home/ubuntu/data/nerf_example_data/nerf_synthetic/lego',
- help='root directory of dataset')
- parser.add_argument('--dataset_name', type=str, default='blender',
- choices=['blender', 'llff'],
- help='which dataset to validate')
- parser.add_argument('--scene_name', type=str, default='test',
- help='scene name, used as output ply filename')
- parser.add_argument('--img_wh', nargs="+", type=int, default=[800, 800],
- help='resolution (img_w, img_h) of the image')
-
- parser.add_argument('--N_samples', type=int, default=64,
- help='number of samples to infer the acculmulated opacity')
- parser.add_argument('--chunk', type=int, default=32*1024,
- help='chunk size to split the input to avoid OOM')
- parser.add_argument('--ckpt_path', type=str, required=True,
- help='pretrained checkpoint path to load')
-
- parser.add_argument('--N_grid', type=int, default=256,
- help='size of the grid on 1 side, larger=higher resolution')
- parser.add_argument('--x_range', nargs="+", type=float, default=[-1.0, 1.0],
- help='x range of the object')
- parser.add_argument('--y_range', nargs="+", type=float, default=[-1.0, 1.0],
- help='x range of the object')
- parser.add_argument('--z_range', nargs="+", type=float, default=[-1.0, 1.0],
- help='x range of the object')
- parser.add_argument('--sigma_threshold', type=float, default=20.0,
- help='threshold to consider a location is occupied')
- parser.add_argument('--occ_threshold', type=float, default=0.2,
- help='''threshold to consider a vertex is occluded.
- larger=fewer occluded pixels''')
-
- #### method using vertex normals ####
- parser.add_argument('--use_vertex_normal', action="store_true",
- help='use vertex normals to compute color')
- parser.add_argument('--N_importance', type=int, default=64,
- help='number of fine samples to infer the acculmulated opacity')
- parser.add_argument('--near_t', type=float, default=1.0,
- help='the near bound factor to start the ray')
-
- return parser.parse_args()
-
-
-@torch.no_grad()
-def f(models, embeddings, rays, N_samples, N_importance, chunk, white_back):
- """Do batched inference on rays using chunk."""
- B = rays.shape[0]
- results = defaultdict(list)
- for i in range(0, B, chunk):
- rendered_ray_chunks = \
- render_rays(models,
- embeddings,
- rays[i:i+chunk],
- N_samples,
- False,
- 0,
- 0,
- N_importance,
- chunk,
- white_back,
- test_time=True)
-
- for k, v in rendered_ray_chunks.items():
- results[k] += [v]
-
- for k, v in results.items():
- results[k] = torch.cat(v, 0)
- return results
-
-
-if __name__ == "__main__":
- args = get_opts()
-
- kwargs = {'root_dir': args.root_dir,
- 'img_wh': tuple(args.img_wh)}
- if args.dataset_name == 'llff':
- kwargs['spheric_poses'] = True
- kwargs['split'] = 'test'
- else:
- kwargs['split'] = 'train'
- dataset = dataset_dict[args.dataset_name](**kwargs)
-
- embedding_xyz = Embedding(3, 10)
- embedding_dir = Embedding(3, 4)
- embeddings = {'xyz': embedding_xyz, 'dir': embedding_dir}
- nerf_fine = NeRF()
- load_ckpt(nerf_fine, args.ckpt_path, model_name='nerf_fine')
- nerf_fine.cuda().eval()
-
- # define the dense grid for query
- N = args.N_grid
- xmin, xmax = args.x_range
- ymin, ymax = args.y_range
- zmin, zmax = args.z_range
- # assert xmax-xmin == ymax-ymin == zmax-zmin, 'the ranges must have the same length!'
- x = np.linspace(xmin, xmax, N)
- y = np.linspace(ymin, ymax, N)
- z = np.linspace(zmin, zmax, N)
-
- xyz_ = torch.FloatTensor(np.stack(np.meshgrid(x, y, z), -1).reshape(-1, 3)).cuda()
- dir_ = torch.zeros_like(xyz_).cuda()
- # sigma is independent of direction, so any value here will produce the same result
-
- # predict sigma (occupancy) for each grid location
- print('Predicting occupancy ...')
- with torch.no_grad():
- B = xyz_.shape[0]
- out_chunks = []
- for i in tqdm(range(0, B, args.chunk)):
- xyz_embedded = embedding_xyz(xyz_[i:i+args.chunk]) # (N, embed_xyz_channels)
- dir_embedded = embedding_dir(dir_[i:i+args.chunk]) # (N, embed_dir_channels)
- xyzdir_embedded = torch.cat([xyz_embedded, dir_embedded], 1)
- out_chunks += [nerf_fine(xyzdir_embedded)]
- rgbsigma = torch.cat(out_chunks, 0)
-
- sigma = rgbsigma[:, -1].cpu().numpy()
- sigma = np.maximum(sigma, 0).reshape(N, N, N)
-
- # perform marching cube algorithm to retrieve vertices and triangle mesh
- print('Extracting mesh ...')
- vertices, triangles = mcubes.marching_cubes(sigma, args.sigma_threshold)
-
- ##### Until mesh extraction here, it is the same as the original repo. ######
-
- vertices_ = (vertices/N).astype(np.float32)
- ## invert x and y coordinates (WHY? maybe because of the marching cubes algo)
- x_ = (ymax-ymin) * vertices_[:, 1] + ymin
- y_ = (xmax-xmin) * vertices_[:, 0] + xmin
- vertices_[:, 0] = x_
- vertices_[:, 1] = y_
- vertices_[:, 2] = (zmax-zmin) * vertices_[:, 2] + zmin
- vertices_.dtype = [('x', 'f4'), ('y', 'f4'), ('z', 'f4')]
-
- face = np.empty(len(triangles), dtype=[('vertex_indices', 'i4', (3,))])
- face['vertex_indices'] = triangles
-
- PlyData([PlyElement.describe(vertices_[:, 0], 'vertex'),
- PlyElement.describe(face, 'face')]).write(f'{args.scene_name}.ply')
-
- # remove noise in the mesh by keeping only the biggest cluster
- print('Removing noise ...')
- mesh = o3d.io.read_triangle_mesh(f"{args.scene_name}.ply")
- idxs, count, _ = mesh.cluster_connected_triangles()
- max_cluster_idx = np.argmax(count)
- triangles_to_remove = [i for i in range(len(face)) if idxs[i] != max_cluster_idx]
- mesh.remove_triangles_by_index(triangles_to_remove)
- mesh.remove_unreferenced_vertices()
- print(f'Mesh has {len(mesh.vertices)/1e6:.2f} M vertices and {len(mesh.triangles)/1e6:.2f} M faces.')
-
- vertices_ = np.asarray(mesh.vertices).astype(np.float32)
- triangles = np.asarray(mesh.triangles)
-
- # perform color prediction
- # Step 0. define constants (image width, height and intrinsics)
- W, H = args.img_wh
- K = np.array([[dataset.focal, 0, W/2],
- [0, dataset.focal, H/2],
- [0, 0, 1]]).astype(np.float32)
-
- # Step 1. transform vertices into world coordinate
- N_vertices = len(vertices_)
- vertices_homo = np.concatenate([vertices_, np.ones((N_vertices, 1))], 1) # (N, 4)
-
- if args.use_vertex_normal: ## use normal vector method as suggested by the author.
- ## see https://github.com/bmild/nerf/issues/44
- mesh.compute_vertex_normals()
- rays_d = torch.FloatTensor(np.asarray(mesh.vertex_normals))
- near = dataset.bounds.min() * torch.ones_like(rays_d[:, :1])
- far = dataset.bounds.max() * torch.ones_like(rays_d[:, :1])
- rays_o = torch.FloatTensor(vertices_) - rays_d * near * args.near_t
-
- nerf_coarse = NeRF()
- load_ckpt(nerf_coarse, args.ckpt_path, model_name='nerf_coarse')
- nerf_coarse.cuda().eval()
-
- results = f({'coarse': nerf_coarse, 'fine': nerf_fine}, embeddings,
- torch.cat([rays_o, rays_d, near, far], 1).cuda(),
- args.N_samples,
- args.N_importance,
- args.chunk,
- dataset.white_back)
-
- else: ## use my color average method. see README_mesh.md
- ## buffers to store the final averaged color
- non_occluded_sum = np.zeros((N_vertices, 1))
- v_color_sum = np.zeros((N_vertices, 3))
-
- # Step 2. project the vertices onto each training image to infer the color
- print('Fusing colors ...')
- for idx in tqdm(range(len(dataset.image_paths))):
- ## read image of this pose
- image = cv2.imread(dataset.image_paths[idx])[:,:,::-1]
- image = cv2.resize(image, tuple(args.img_wh))
-
- ## read the camera to world relative pose
- P_c2w = np.concatenate([dataset.poses[idx], np.array([0, 0, 0, 1]).reshape(1, 4)], 0)
- P_w2c = np.linalg.inv(P_c2w)[:3] # (3, 4)
- ## project vertices from world coordinate to camera coordinate
- vertices_cam = (P_w2c @ vertices_homo.T) # (3, N) in "right up back"
- vertices_cam[1:] *= -1 # (3, N) in "right down forward"
- ## project vertices from camera coordinate to pixel coordinate
- vertices_image = (K @ vertices_cam).T # (N, 3)
- depth = vertices_image[:, -1:]+1e-5 # the depth of the vertices, used as far plane
- vertices_image = vertices_image[:, :2]/depth
- vertices_image = vertices_image.astype(np.float32)
- vertices_image[:, 0] = np.clip(vertices_image[:, 0], 0, W-1)
- vertices_image[:, 1] = np.clip(vertices_image[:, 1], 0, H-1)
-
- ## compute the color on these projected pixel coordinates
- ## using bilinear interpolation.
- ## NOTE: opencv's implementation has a size limit of 32768 pixels per side,
- ## so we split the input into chunks.
- colors = []
- remap_chunk = int(3e4)
- for i in range(0, N_vertices, remap_chunk):
- colors += [cv2.remap(image,
- vertices_image[i:i+remap_chunk, 0],
- vertices_image[i:i+remap_chunk, 1],
- interpolation=cv2.INTER_LINEAR)[:, 0]]
- colors = np.vstack(colors) # (N_vertices, 3)
-
- ## predict occlusion of each vertex
- ## we leverage the concept of NeRF by constructing rays coming out from the camera
- ## and hitting each vertex; by computing the accumulated opacity along this path,
- ## we can know if the vertex is occluded or not.
- ## for vertices that appear to be occluded from every input view, we make the
- ## assumption that its color is the same as its neighbors that are facing our side.
- ## (think of a surface with one side facing us: we assume the other side has the same color)
-
- ## ray's origin is camera origin
- rays_o = torch.FloatTensor(dataset.poses[idx][:, -1]).expand(N_vertices, 3)
- ## ray's direction is the vector pointing from camera origin to the vertices
- rays_d = torch.FloatTensor(vertices_) - rays_o # (N_vertices, 3)
- rays_d = rays_d / torch.norm(rays_d, dim=-1, keepdim=True)
- near = dataset.bounds.min() * torch.ones_like(rays_o[:, :1])
- ## the far plane is the depth of the vertices, since what we want is the accumulated
- ## opacity along the path from camera origin to the vertices
- far = torch.FloatTensor(depth) * torch.ones_like(rays_o[:, :1])
- results = f({'coarse': nerf_fine}, embeddings,
- torch.cat([rays_o, rays_d, near, far], 1).cuda(),
- args.N_samples,
- 0,
- args.chunk,
- dataset.white_back)
- opacity = results['opacity_coarse'].cpu().numpy()[:, np.newaxis] # (N_vertices, 1)
- opacity = np.nan_to_num(opacity, 1)
-
- non_occluded = np.ones_like(non_occluded_sum) * 0.1/depth # weight by inverse depth
- # near=more confident in color
- non_occluded += opacity < args.occ_threshold
-
- v_color_sum += colors * non_occluded
- non_occluded_sum += non_occluded
-
- # Step 3. combine the output and write to file
- if args.use_vertex_normal:
- v_colors = results['rgb_fine'].cpu().numpy() * 255.0
- else: ## the combined color is the average color among all views
- v_colors = v_color_sum/non_occluded_sum
- v_colors = v_colors.astype(np.uint8)
- v_colors.dtype = [('red', 'u1'), ('green', 'u1'), ('blue', 'u1')]
- vertices_.dtype = [('x', 'f4'), ('y', 'f4'), ('z', 'f4')]
- vertex_all = np.empty(N_vertices, vertices_.dtype.descr+v_colors.dtype.descr)
- for prop in vertices_.dtype.names:
- vertex_all[prop] = vertices_[prop][:, 0]
- for prop in v_colors.dtype.names:
- vertex_all[prop] = v_colors[prop][:, 0]
-
- face = np.empty(len(triangles), dtype=[('vertex_indices', 'i4', (3,))])
- face['vertex_indices'] = triangles
-
- PlyData([PlyElement.describe(vertex_all, 'vertex'),
- PlyElement.describe(face, 'face')]).write(f'{args.scene_name}.ply')
-
- print('Done!')
diff --git a/extract_mesh.ipynb b/extract_mesh.ipynb
deleted file mode 100644
index b02e2a01..00000000
--- a/extract_mesh.ipynb
+++ /dev/null
@@ -1,252 +0,0 @@
-{
- "cells": [
- {
- "cell_type": "code",
- "execution_count": 1,
- "metadata": {},
- "outputs": [],
- "source": [
- "import torch\n",
- "from collections import defaultdict\n",
- "import numpy as np\n",
- "import mcubes\n",
- "import trimesh\n",
- "\n",
- "from models.rendering import *\n",
- "from models.nerf import *\n",
- "\n",
- "from datasets import dataset_dict\n",
- "\n",
- "from utils import load_ckpt"
- ]
- },
- {
- "cell_type": "markdown",
- "metadata": {},
- "source": [
- "# Load model and data"
- ]
- },
- {
- "cell_type": "code",
- "execution_count": 2,
- "metadata": {},
- "outputs": [],
- "source": [
- "# Change here #\n",
- "img_wh = (1216, 1622) # full resolution of the input images\n",
- "dataset_name = 'llff' # blender or llff (own data)\n",
- "scene_name = 'jenny1' # whatever you want\n",
- "root_dir = '/home/ubuntu/data/nerf_example_data/my/jenny1/' # the folder containing data\n",
- "ckpt_path = 'ckpts/jenny1/epoch=35.ckpt' # the model path\n",
- "###############\n",
- "\n",
- "kwargs = {'root_dir': root_dir,\n",
- " 'img_wh': img_wh}\n",
- "if dataset_name == 'llff':\n",
- " kwargs['spheric_poses'] = True\n",
- " kwargs['split'] = 'test'\n",
- "else:\n",
- " kwargs['split'] = 'train'\n",
- " \n",
- "chunk = 1024*32\n",
- "dataset = dataset_dict[dataset_name](**kwargs)\n",
- "\n",
- "embedding_xyz = Embedding(3, 10)\n",
- "embedding_dir = Embedding(3, 4)\n",
- "\n",
- "nerf_fine = NeRF()\n",
- "load_ckpt(nerf_fine, ckpt_path, model_name='nerf_fine')\n",
- "nerf_fine.cuda().eval();"
- ]
- },
- {
- "cell_type": "markdown",
- "metadata": {},
- "source": [
- "# Search for tight bounds of the object (trial and error!)"
- ]
- },
- {
- "cell_type": "code",
- "execution_count": null,
- "metadata": {},
- "outputs": [],
- "source": [
- "### Tune these parameters until the whole object lies tightly in range with little noise ###\n",
- "N = 128 # controls the resolution, set this number small here because we're only finding\n",
- " # good ranges here, not yet for mesh reconstruction; we can set this number high\n",
- " # when it comes to final reconstruction.\n",
- "xmin, xmax = -0.5, 0.6 # left/right range\n",
- "ymin, ymax = -0.5, 0.6 # forward/backward range\n",
- "zmin, zmax = -2., -0.9 # up/down range\n",
- "## Attention! the ranges MUST have the same length!\n",
- "sigma_threshold = 5. # controls the noise (lower=maybe more noise; higher=some mesh might be missing)\n",
- "############################################################################################\n",
- "\n",
- "x = np.linspace(xmin, xmax, N)\n",
- "y = np.linspace(ymin, ymax, N)\n",
- "z = np.linspace(zmin, zmax, N)\n",
- "\n",
- "xyz_ = torch.FloatTensor(np.stack(np.meshgrid(x, y, z), -1).reshape(-1, 3)).cuda()\n",
- "dir_ = torch.zeros_like(xyz_).cuda()\n",
- "\n",
- "with torch.no_grad():\n",
- " B = xyz_.shape[0]\n",
- " out_chunks = []\n",
- " for i in range(0, B, chunk):\n",
- " xyz_embedded = embedding_xyz(xyz_[i:i+chunk]) # (N, embed_xyz_channels)\n",
- " dir_embedded = embedding_dir(dir_[i:i+chunk]) # (N, embed_dir_channels)\n",
- " xyzdir_embedded = torch.cat([xyz_embedded, dir_embedded], 1)\n",
- " out_chunks += [nerf_fine(xyzdir_embedded)]\n",
- " rgbsigma = torch.cat(out_chunks, 0)\n",
- " \n",
- "sigma = rgbsigma[:, -1].cpu().numpy()\n",
- "sigma = np.maximum(sigma, 0)\n",
- "sigma = sigma.reshape(N, N, N)\n",
- "\n",
- "# The below lines are for visualization, COMMENT OUT once you find the best range and increase N!\n",
- "vertices, triangles = mcubes.marching_cubes(sigma, sigma_threshold)\n",
- "mesh = trimesh.Trimesh(vertices/N, triangles)\n",
- "mesh.show()"
- ]
- },
- {
- "cell_type": "code",
- "execution_count": null,
- "metadata": {},
- "outputs": [],
- "source": [
- "# # You can already export \"colorless\" mesh if you don't need color\n",
- "# mcubes.export_mesh(vertices, triangles, f\"{scene_name}.dae\")"
- ]
- },
- {
- "cell_type": "markdown",
- "metadata": {},
- "source": [
- "# Generate .vol file for volume rendering in Unity"
- ]
- },
- {
- "cell_type": "code",
- "execution_count": null,
- "metadata": {},
- "outputs": [],
- "source": [
- "assert N==512, \\\n",
- " 'Please set N to 512 in the two above cell! Remember to comment out the visualization code (last 3 lines)!'\n",
- "\n",
- "a = 1-np.exp(-(xmax-xmin)/N*sigma)\n",
- "a = a.flatten()\n",
- "rgb = (rgbsigma[:, :3].numpy()*255).astype(np.uint32)\n",
- "i = np.where(a>0)[0] # valid indices (alpha>0)\n",
- "\n",
- "rgb = rgb[i]\n",
- "a = a[i]\n",
- "s = rgb.dot(np.array([1<<24, 1<<16, 1<<8])) + (a*255).astype(np.uint32)\n",
- "res = np.stack([i, s], -1).astype(np.uint32).flatten()\n",
- "with open(f'{scene_name}.vol', 'wb') as f:\n",
- " f.write(res.tobytes())"
- ]
- },
- {
- "cell_type": "markdown",
- "metadata": {},
- "source": [
- "# Extract colored mesh\n",
- "\n",
- "Once you find the best range, now **RESTART** the notebook, and copy the configs to the following cell\n",
- "and execute it."
- ]
- },
- {
- "cell_type": "code",
- "execution_count": 3,
- "metadata": {},
- "outputs": [
- {
- "name": "stdout",
- "output_type": "stream",
- "text": [
- "Predicting occupancy ...\n",
- "100%|████████████████████████████████████████| 512/512 [00:03<00:00, 159.97it/s]\n",
- "Extracting mesh ...\n",
- "Removing noise ...\n",
- "Mesh has 0.32 M vertices and 0.63 M faces.\n",
- "Fusing colors ...\n",
- "100%|███████████████████████████████████████████| 20/20 [01:06<00:00, 3.32s/it]\n",
- "Done!\n"
- ]
- }
- ],
- "source": [
- "# Copy the variables you have above here! ####\n",
- "img_wh = (1216, 1622) # full resolution of the input images\n",
- "dataset_name = 'llff' # blender or llff (own data)\n",
- "scene_name = 'jenny1' # whatever you want\n",
- "root_dir = '/home/ubuntu/data/nerf_example_data/my/jenny1/' # the folder containing data\n",
- "ckpt_path = 'ckpts/jenny1/epoch=35.ckpt' # the model path\n",
- "\n",
- "N = 128 # controls the resolution, set this number small here because we're only finding\n",
- " # good ranges here, not yet for mesh reconstruction; we can set this number high\n",
- " # when it comes to final reconstruction.\n",
- "xmin, xmax = -0.5, 0.6 # left/right range\n",
- "ymin, ymax = -0.5, 0.6 # forward/backward range\n",
- "zmin, zmax = -2, -0.9 # up/down range\n",
- "## Attention! the ranges MUST have the same length!\n",
- "sigma_threshold = 5. # controls the noise (lower=maybe more noise; higher=some mesh might be missing)\n",
- "###############################################\n",
- "\n",
- "import os\n",
- "os.environ['ROOT_DIR'] = root_dir\n",
- "os.environ['DATASET_NAME'] = dataset_name\n",
- "os.environ['SCENE_NAME'] = scene_name\n",
- "os.environ['IMG_SIZE'] = f\"{img_wh[0]} {img_wh[1]}\"\n",
- "os.environ['CKPT_PATH'] = ckpt_path\n",
- "os.environ['N_GRID'] = \"256\" # final resolution. You can set this number high to preserve more details\n",
- "os.environ['X_RANGE'] = f\"{xmin} {xmax}\"\n",
- "os.environ['Y_RANGE'] = f\"{ymin} {ymax}\"\n",
- "os.environ['Z_RANGE'] = f\"{zmin} {zmax}\"\n",
- "os.environ['SIGMA_THRESHOLD'] = str(sigma_threshold)\n",
- "os.environ['OCC_THRESHOLD'] = \"0.2\" # probably doesn't require tuning. If you find the color is not close\n",
- " # to real, try to set this number smaller (the effect of this number\n",
- " # is explained in my youtube video)\n",
- "\n",
- "!python extract_color_mesh.py \\\n",
- " --root_dir $ROOT_DIR \\\n",
- " --dataset_name $DATASET_NAME \\\n",
- " --scene_name $SCENE_NAME \\\n",
- " --img_wh $IMG_SIZE \\\n",
- " --ckpt_path $CKPT_PATH \\\n",
- " --N_grid $N_GRID \\\n",
- " --x_range $X_RANGE \\\n",
- " --y_range $Y_RANGE \\\n",
- " --z_range $Z_RANGE \\\n",
- " --sigma_threshold $SIGMA_THRESHOLD \\\n",
- " --occ_threshold $OCC_THRESHOLD"
- ]
- }
- ],
- "metadata": {
- "kernelspec": {
- "display_name": "nerf_pl",
- "language": "python",
- "name": "nerf_pl"
- },
- "language_info": {
- "codemirror_mode": {
- "name": "ipython",
- "version": 3
- },
- "file_extension": ".py",
- "mimetype": "text/x-python",
- "name": "python",
- "nbconvert_exporter": "python",
- "pygments_lexer": "ipython3",
- "version": "3.6.12"
- }
- },
- "nbformat": 4,
- "nbformat_minor": 4
-}